The Three Pillars of Modern Transaction Monitoring
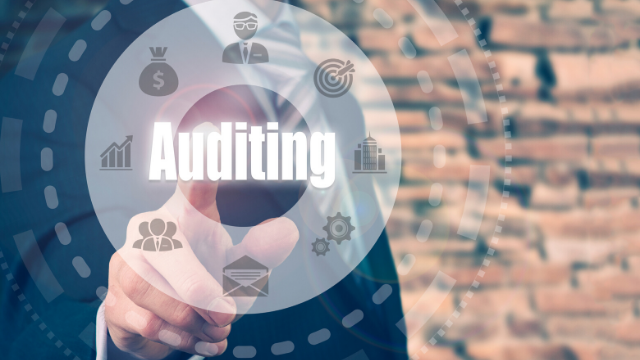
Transaction monitoring – the cornerstone of the audit process
It has been a way to infer to some level of probability that a control is effective. The control of greatest significance for the longest time were the accounts with their own magical double-entry controls. Confirming the validity of balances, allowed an auditor to express whether they represent a true and fair view of the financial affairs of the company. This in turn allows an investor to judge the amounts, timing, and certainty of future cash flows. It coincidentally has some capability to find fraud. Now we all realize that by the time an issue is reflected in the books of account, any issue is by definition historical with little chance to correct. We have controls in place within processes that are far upstream from the accounts. We also all realize that we have obligations to constituencies beyond investors: employees, customers, vendors, and that controls must be in place to protect their interests. Of course, we now have legislation that enforces these obligations, not least of which is GDPR. We also realize that damage can be done to a company’s reputation even if the internal controls over financial reporting are effective. For all these reasons transactions need to be monitored to confirm that internal controls, financial, information security and reputation are well designed and remain effective.
Sampling
Within the Auditors toolkit has always been sampling, as a way to predict with a known level of probability whether the hypothesis that a control is effective can be asserted. Verifying every transaction by human power is an expense rarely justifiable to shareholders. Even sampling has had some improvements with the ability to coordinate sample sizing with control frequency and coordinate samples with audit procedures, records request and engagement letters. In this way, samples can support the evaluation of many controls in the overall audit plan. With the early focus on the accounts as being the touchstone of internal controls, the hypothesis being tested was that every balance could be supported by underlying transactions and that all transactions get processed. As the audit universe has expanded, hypotheses being tested have expanded: all authorizations have been approved, all expense claims are legitimate, all employees have passed background checks, all activity on the network is legitimate.
Continuous Controls Monitoring
With the need to increase the reliability of controls, coupled with the need to make the control verification methodologies much more efficient, transaction monitoring moved into the realm of rules being applied and every transaction being evaluated. If we take the example of all expense reports being legitimate, we may have rules that report numerous transactions just under authorization limit or multiple employees with expenses to the same vendor on the same day. Being able to report these allows us to move from a sample-based approach to a substantive approach and therefore have a much higher degree of confidence in the assertion. This increase has only been viable because of the ability to deploy specialist tools for continuous controls monitoring, but they can only be as good as the rules that they evaluate.
Machine Learning
Expressing rules in Neural Net and Structured Queries.
We are all very familiar with a rule as being expressed in a structured query language. For example, if you are looking at general ledger transactions, transactions that release reserves very close to a reporting date might be subject to harsher review for their ability to arbitrarily move a profit number. We can envisage a very simple select statement that might locate those transactions. Similarly, if we are seeing large outbound data volumes from the network, we should be suspicious of the activity and we can see how we might select those from a log. These can also be represented as relationships between attributes in a neural network. The relationships between different attributes may be strong with relationship to a particular outcome. In this case, the outcome is that a transaction is worthy of investigation. We can use our assessment of current transactions as to whether they are worthy of investigation, to strengthen and weaken the relationships. For example, a release of reserves within 3 days of reporting may be flagged strengthening the relationship, and another release of reserves within 5 days may not be flagged weakening the relationship. Of course, this requires considerable transformation and manipulation from the originating system.
Absorbing Findings and Observations back into the rules
As we gather findings and observations within audit engagements we will also be strengthening and weakening the relationships further refining the model.
As we evaluation transactions that have been flagged in continuous transaction monitoring they are also ploughed back into the model, strengthening or weakening the assumptions between attributes.
Once we have absorbed our findings and observations, the result of what attributes and their values are primary indicators of a problem, can be expressed in the structured query language that is more humanly readable.
Finding Anomalies and Outliers
Even in a well-tuned neural model, it is still increasing and decreasing the relationships between the features that are inherent in the model. It is still important to analyze the data for patterns and outliers that are not yet taken into consideration in the model. In the example above with reserves being released, we may find that transactions from reserves are much more likely to happen on a particular day of the week because a supervisor is absent. We may find the purchasing transactions “off contract” are concentrated on a particular vendor. We can use standard statistical methods to find clusters and anomalies and work these features back into our neural network model.
Where is Transaction Monitoring going?
Audit as a Service and mining for audit rules
Anomalous transactions are by definition rare. The rate of learning is very dependant on having training data. SaaS companies have access to the precious commodity of fraudulent transactions across their tenants. This means members of the service share the spoils of the service in terms of the refinement of the rules. It also means that the service provider has to be able to guarantee privacy in the use of the learning data. Extraction and Anonymization capabilities must be transparent so that all parties can understand this risk.
Audit Optimisation
Given that manual audit will continue to be necessary we need to develop an audit program that will focus scarce audit resources on where they can provide the most value to the organization. Objective Function to minimize residual risk, maximize reliability, maximize confidence in the assertion and minimize cost, subject to the constraints of limited people and budgets. Audit Procedures confirm a set of controls that if they prove to be effective, reduce the residual risk in a number of risks identified in the risk assessment phase of an audit program. We also know the time and costs associated with their execution. This allows us to ranks them according to their “risk removed”. We can also modify the time and cost for sample size in the control and increase or decrease the confidence in the result. In this way we can optimize for both “Risk removed” and “Confidence Gained” subject to cost and resource constraints.
Conclusion
A transaction monitoring solution is vital to protect the validity of financial reporting, assets of the enterprise, Information security, and reputation of any organization. Transaction monitoring is necessary to confirm internal controls are designed well and working effectively. Having adequate internal controls is mandated under many regulations that now include GDPR. A transaction monitoring solution should include manual audit, continuous transaction monitoring machine learning and audit optimization. Specialist providers in the cloud have an advantage in speed of learning through getting training data from many tenants.